29 September 2020
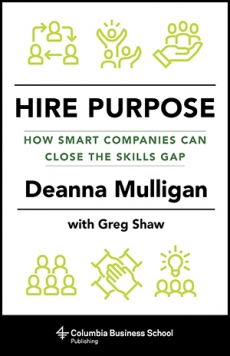
Hire Purpose: How Smart Companies Can Close the Skills Gap
Deanna Mulligan
280 pages, Columbia Business School Publishing, 2020
Tech companies and their customers report that the coronavirus crisis has significantly accelerated digital transformations across the public and private sectors. Remote work, virtual reality, and artificial intelligence are gaining usage at lightning speed. This means the urgency of re-skilling and upskilling the workforce has also intensified. That challenge is complex. In her new book, Hire Purpose: How Smart Companies Can Close the Skills Gap, Guardian Life Insurance CEO Deanna Mulligan explains how even her industry’s ancient practice of actuarial science has had to retrain using next-generation skills, and how society must now do the same.—Deanna Mulligan
****
Early in my tenure as CEO, I asked our team at Guardian to become experts in the future of work and how workers can acquire the necessary skills to succeed. We want our people and our policyholders alike to be high achievers for current jobs and for long-term career opportunities. We also want to meet the needs of local economies and society.
Toward these ends, we chose to invest significantly in better understanding two areas in the field of workforce development: bridging the skills gap and preparing for digital convergence. On the skills front, people need communications and collaboration skills as well as a baseline of technology skills. On the digital convergence side, they need to unify technology and business planning. They need to be data savvy.
Nowhere is that more poignant than among our own actuaries, the professionals who calculate insurance and annuity premiums, reserves, and dividends. Manufacturers have engineers, hospitals have doctors, and law firms have attorneys. Those who surround and support them are essential, but they are at the core of the business. Insurance companies rely on actuaries.
They are often portrayed in popular culture as accountants in green eyeshades, staring at numbers and equations beneath the light of a desk lamp. Ben Stiller, Fred MacMurray, Jack Nicholson, and Edward Norton have played actuaries in Hollywood. Comedian Will Farrell played an IRS auditor whose fiancé left him for an actuary – adding insult to injury. In Batman, a mathematical genius named The Actuary was drawn as a villain who applies his mathematical formulas to aid the Penguin.
Actuaries, in fact, are heroes who will only add superpowers with the addition of data science, machine learning, and artificial intelligence. The future of actuarial sciences will become more precise over time and as we reason over more data. Assessing one person’s health, for example, is easier the more data you have and the more patterns you recognize. AI will improve our fraud detection and claims management process. It will make more effective and efficient the 150,000 emails per year we receive requesting rate quotes from agents and brokers. It will improve how we determine risk and evaluate pricing.
Guardian’s Chief Actuary, Michael Slipowitz, discovered his love of numbers and math while following his favorite sports teams, notably the New York Yankees. Every day he poured over the statistics on the sports page in the local newspaper. Noticing this inclination, his mother suggested he look at the actuarial profession as his baseball talents waned and he started to think about what he wanted to do when he grew up. He followed a well-worn trail to becoming an actuary, studying accounting, joining an insurance company, taking his exams, and being admitted to the Society of Actuaries. He rose through the ranks by solving increasingly complex problems and questions. What is the propensity of a group to buy one or more insurance products? What is the propensity of policyholders to retain their policies? What is the correlation between those who go to the dentist twice a year and lifespan? Actuaries examine investments and risk. What if a pandemic struck and an unpredictable number of insurance claims were filed?
Actuaries have long answered these and even more complex questions with a high degree of confidence, but data science and its many branches will increasingly help us to answer them with greater precision. To do so, however, we need people with hybrid skills. Actuaries with insurance industry expertise who also know how to use programming languages like Python to explore data for answers. Investment people who know financial markets but can also take structured and unstructured data to identify trends and valuation patterns.
Actuaries have grown up around a wide range of insurance instruments, including social security, Medicare, and Medicaid. Continuous education and technology are intertwined with the profession. For actuaries, as it should be for all professions, data science will advance the field. At Guardian, we process tens of millions of claims. No one person can manage that, but data science and AI are showing promising results. In the past, actuaries might analyze several variables in order to estimate a likely outcome. Today we see the potential to leverage an exponential amount of data and variables It’s a whole new layer of complexity. Clearly, the skills of the actuary—like those we all possess—must evolve.
A parlor game in some tech circles is to question the future of the radiologist. What is to become of this profession if the machine can diagnose an x-ray faster and more accurately than a human radiologist? That’s a parlor game that is expanding to include more and more professions. Will actuaries become data scientists? Will data scientists be replaced by self-created algorithms and AI technologies?
A Brookings Institute in November 2019 published a study examining new AI-related patents and job descriptions to interpret which jobs and which cities will be most impacted by digital disruption. The radiologist was among them but so were carmakers and clothiers, purchasing agents and agricultural workers. The Axios headline proclaimed: AI is coming for white-collar and blue-collar workers.1
In my view, artificial intelligence in all its forms—machine learning, deep neural networks, natural language processing, visual recognition—will augment rather than replace our human skills and capacities. The new actuary needs to be steeped in large quantities of data and the opportunity is that some of these new data sources could make accessing insurance easier. Insurance, like more and more businesses, is a data company at the core. We have to hire for and train people to manage asymmetric data better than others.
Tom Olds, a member of my leadership team, is a good example of the asymmetric skillset we need. Today he leads predictive analytics for Guardian, and his team includes both data scientists and a number of physicists, including an astrophysicist. Tom explains that actuarial science and computer science are similar – they both use continually increasing data sets to ascertain “signal to noise” – that is to say the ratio of “signal,” or insights and predictions to “noise,” or unwanted background. Tom sees the merging of data, actuarial science, machine learning and artificial intelligence as a certainty that will lead to better insights, better health and better insurance planning.
One of Tom’s physicist-turned-actuary is Yun Wang, who trained at Zhejiang University in China. His story illustrates the journey toward precisions insurance. Ryan, as he prefers to be called, went on to study at Washington University in St. Louis, where he worked on a National Institutes of Health (NIH) grant to develop sensors that can better target drugs. The project brought together students from medicine, chemistry, physics and engineering. There he was introduced to pattern recognition using algorithms and early instances of machine learning. During lab time friends would talk about Wall Street and how actuarial science was being used to assess risk and opportunity. It captured his imagination but he was turned off by what he saw as greed and instead decided to head for Hartford, Connecticut, a mecca for the insurance industry, to study actuarial science at the University Connecticut. He immediately recognized the math—floating point, partial differential equations and advanced stats – as similar to physics.
Today Ryan is studying patterns with machine learning and AI to root out fraudulent claims, which cost the industry and consumers billions of dollars. He’s also using data to improve risk assessment for underwriting life insurance. Millennials are now the future policyholders and they expect the process of buying life insurance to be quick and painless. AI is shortening the process from an average of 32 days to just 8. AI also will gradually reduce the need for medical tests like blood and urine samples.
It will become exceedingly difficult to compete in the marketplace and for individual job prospects to compete for work without some familiarity with these digital tools. Businesses, industries, and people will be disrupted. Erik Brynjolfsson and Andrew McAfee, authors of The Second Machine Age, are optimistic about the prospects of digital transformation, but also warn that it will bring thorny challenges. “Technological progress is going to leave behind some people, perhaps a lot of people, as it races ahead.”
The routine things humans do, repeatable functions, will be more easily replaced than higher-level, skilled functions. But machines, equipped with deep neural networks, agents and bots, will also become intelligent learners. As Brynjolfsson and McAfee conclude: there’s never been a better time to be a worker with special skills and the right education, or a worse time to be a worker with only “ordinary” skills and abilities.
As insurance professionals, we must care about digital transformation—a lot. We need to become experts at understanding labor and education trends, both at the client level and at the macroeconomic level. If we are slow to understand how people and their work are changing, we will be slow to understand health outcomes and morbidity.
Nobel Prize winning economist Angus Deaton and Anne Case have pioneered the link between education and skill levels—which often dictate what jobs are possible—and morbidity.
“We propose a preliminary but plausible story in which cumulative disadvantage from one birth cohort to the next—in the labor market, in marriage and child outcomes, and in health—is triggered by progressively worsening labor market opportunities.”
A case in point has been the gig industry, which before COVID-19 employed as many people as the government sector. Those finding gigs, or time-limited jobs, through ride-sharing services like Uber and Lyft or freelancer.com, are often not eligible for insurance services. Steve King, a partner and co-founder of Emergent Research, estimates there are 18 million full-time gig workers and another 22 million Americans who are part-time.
In their startling book on the future of jobs, Ghost Work, Mary L. Gray and Siddharth Suri write that this gig economy is quietly moving to what they call ghost work on platforms like Amazon’s Mechanical Turk, or MTurk, where “requesters” can post a wide variety of online tasks and workers can perform those tasks – often for a few cents at a time.2 A typical task might be label a series of photos or to test your ability to type in a series of numbers or letters. The World Bank projects these tasks will grow to $25 billion annually by 2020. How people define their jobs and work is clearly changing.
These are people and families, many still pursuing an education, who are struggling economically and need insurance to prevent future emergencies. One in three have an employer sponsored retirement plan, one in four an employer sponsored medical plan, and just one in five have dental, disability, or life insurance. Recognizing there is a new customer to serve, Guardian launched a digital storefront to help protect part-time workers, freelancers, and retirees who do not have access to benefits at work. We also invested in more than a dozen companies to help generate insights into the gig worker.
One investment was in Jobble.com, an online service that can schedule your work day with part-time jobs and tasks, both skilled and unskilled. Education and training matters to the future employment of everyone, regardless of their background. The question becomes what is the best way to prepare for the future and how do we make it accessible to all? How do we avoid one monolithic education model in order to include broad perspectives?
Inspired and informed by our listening and learning within this workforce development community, we’ve developed an approach designed to accomplish a set of outcomes for the next generation of workforce development. Management gurus have their favorite language for these theories – a theory of change, theory of action, change model. I think of it as an alignment of missions and resources that if executed properly could produce both the near- and long-term outcomes we all seek.
In the figure below, we envision individual workers, business leaders, policymakers and educators working together to modernize, strengthen and integrate workforce development by leveraging 3 major public-private investments:
- Education & Training – This is formal, traditional classroom-based approaches in K-12, post-secondary and out of school time at home or with a supplemental tutor.
- Workplace Training and Skills Development – These are more informal settings for education and training including on-the-job.
- Existing Workforce – While the first two sets of investments largely target the emerging workforce, this is aimed at adult education within the existing workforce. It includes life-long learning
In The Knowledge Gap: The Hidden Cause of America’s Broken Education System – and how to fix it – education journalist Natalie Wexler concludes that, “there’s no one right way to provide a high-quality education, and this country is too big and varied for one-size-fits-all prescriptions.” She’s right that we’ll need to simultaneously pursue a range of reform efforts.
Thus, reading from left-to-right, businesses, industry and government can and must partner across education & training, workplace opportunities and programs that target existing workers. Yes, STEM. Yes, grit and growth mindsets. But we must also up our game on tutoring. We need to modernize vo-tech. Yes, internships and apprenticeships. But businesses and industries would be well-served to align job descriptions, especially new jobs like data labelers and data scientists, to ensure that workers – applicants—can prepare for more job opportunities from more companies. If one data center describes a lower-level role one way, and the data center across the road describes it differently – and yet it’s essentially the same job – how does a worker prepare? Yes, adult education and life-long learning, but how can expert partners like General Assembly help to up-skill your workforce? Paid family leave helps to ensure that even if you have the correct skills, you can still have a family and support that family.
Toward a New Equation for Preparing Workers
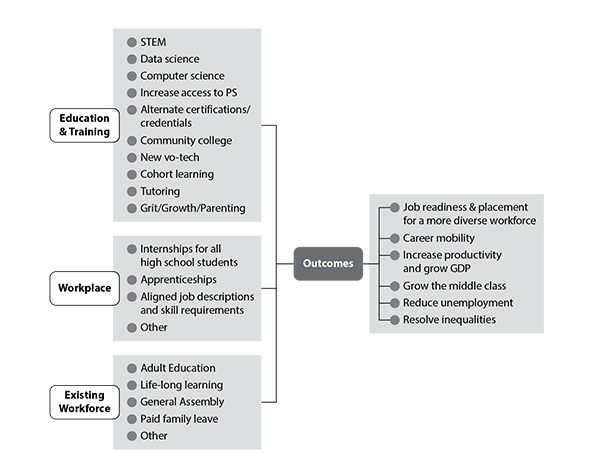
But who should these investments target? How should we allocate limited resources? For purposes of this exercise, I say everyone, but focus on those who need it most. The Haas Institute at the University of California notes that “in an era of political polarization and fiscal austerity, policy debates too readily become trapped in a binary of either universal responses or targeted solutions.” Its report, Targeted Universalism, calls for universal goals pursued by targeted processes to achieve these goals.3
The outcomes, these investments, are designed to drive forward: job readiness and job placement as well as great career mobility, lower unemployment and widening middle class. These outcomes, in turn, promote increased productivity, economic growth and reduced inequality. The barriers, of course, are many, including lack of funding, a fragmented workforce development pipeline, a lack scale and capacity among those most qualified to do the work, which results in a failure to spread evidence-based programs that work.
In his book The Years that Matter Most: How College Makes or Breaks Us, Paul Tough writes that since 2008, state legislators have cut approximately $14 billion in funding from public universities, or approximately 20 percent.
While the vision presented here is ambitious, so was the Higher Education Act of 1965, which has long expired and not been reauthorized. The Act passed both the House and Senate overwhelmingly in 1965 when President Lyndon Johnson was President was amended in 2001 when President George W. Bush was President. Today it presents an opportunity for just the kind of integrated approach proposed here.
Source: ssir.org